QuickDA - Python library for Quick Exploratory Data Analysis
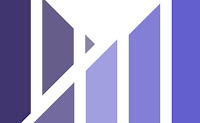
The QuickDA is an open-source EDA tool that helps to build a successful data model from the structured dataset.
Understanding the business model, getting reliable data, and preparing the data for modeling is another huge exercise. It is important to find out what each of the data points signifies.
Most of the time the modeling is failed because of poor insight into data due to improper exploration & analysis. The finest data should be derived only by cleaning the available data before building the model.
Data cleaning is an art. To create the finest art, you need the finest data. Otherwise, it is just a stained one.
Data cleaning is an art. To create the finest art, you need the finest data. Otherwise, it is just a stained one.
There’s an old saying, “garbage in, garbage out”;
Most of the time understanding data demand lots of coding to make it perfect for modeling.
When the data is getting traversed, QuickDA also validates data sanity for high cardinality, high correlation, missing data, uniform data, zeros, and in some cases it automatically corrects the data for better quality.
QuickDA is loaded with libraries to summarize and profiling the dataset, standardizing columns, quickly drop the columns that don’t make sense, remove duplicate rows, replace or drop nulls and missing values, reduce cardinality, build correlation and predictive power score matrix, draw scatter & violin plots, pivot views, explore time series data, etc.
With this EDA tool "QuickDA", you can quickly go from knowing very little about your data to being able to prepare and visualize it with minimum customization.
Happy exploring QuickDA!
QuickDA Resource Center: Pypi Org
QuickDA Blog: Medium
QuickDA Author: Sidheswar Venkatachalapathi
Cheers,
Comments
Post a Comment